Data Lake vs Data Warehouse: What is the Core Difference?
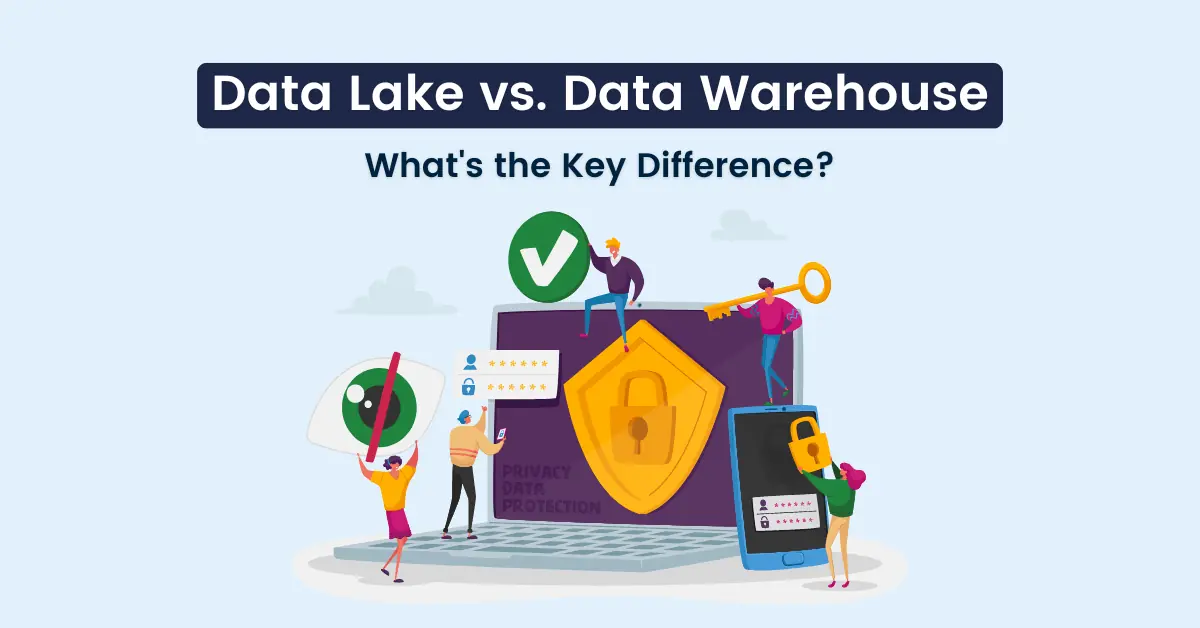
Data is the new oil. That’s the message we hear all the time, but what does it mean? Data is currency. It’s an asset that can be traded and sold in exchange for other assets and services. And like any other asset, you need to protect it—and that means understanding where data comes from, how you use it, how you store it, and most importantly knowing data lake vs data warehouse.
The world of data warehousing is a complex one, with many terms that are often used interchangeably. In this blog, we’ll be going over some of the key differences between these two types of analytical solutions to help you understand what they are, how they work, and why they matter in your own business.
When it comes to knowing the differences between data warehousing and a data lake, it could take time to know where to begin.
What is a Data Warehouse?
A data warehouse is a system that combines data from multiple sources to enable business insights. It is usually used by decision-makers within the organization, like managers or executives.
A data warehouse is different from a data lake in the sense that it has some structure in place while a data lake doesn’t have any specific structure. Data warehouses are used by organizations to store and analyze large amounts of data.
One of the main differences between a data warehouse and a data lake is that a data warehouse consists of historical – old data, whereas the data in a data lake is much more precise and up to the mark.
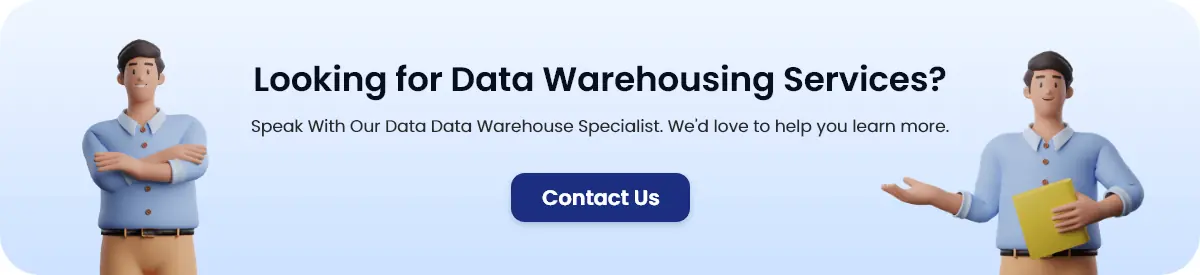
What is a Data Lake?
A data lake is a storage repository that stores raw data from different sources. It is designed to store large volumes of data and supports unstructured, semi-structured, and structured datasets.
In the past, organizations had separate systems for each type of data they collected: a transactional system for business transactions, an ERP (enterprise resource planning) system for financials, and so on; but now they can collect all their data in one location.
This type of architecture allows you to better analyze your entire organization’s operations by aggregating information from various sources into one place and then performing analytics on it.
Key Features of Data Warehouse
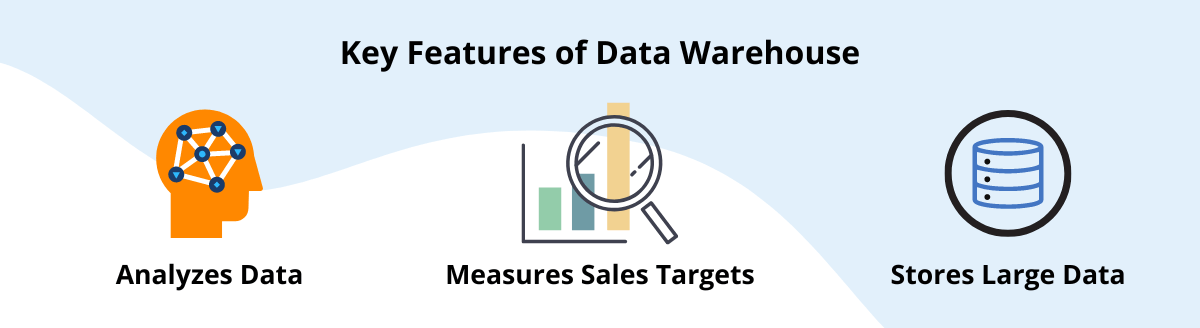
1. Analyzes data
Data warehouses are used to store and analyze data from multiple sources. These can include operational databases, transactional databases, and online analytical processing (OLAP) cubes. They can also be used for decision support, OLAP, and data mining purposes.
2. Measures sales targets
A typical example of this would be when you want to find out how many customers bought your product in the last six months or whether your sales team has been meeting their targets on a weekly basis since January 2019 up until now.
3. Stores large data
Data warehouse can easily store large amounts of data and provide information when needed, rather than regularly.
Top Core Features of Data Lake
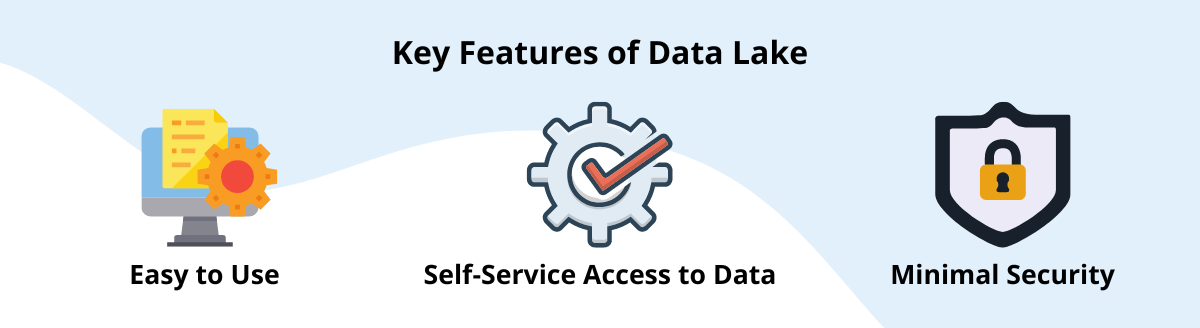
1. Easy to use
Data Lake is easy to use because it uses a self-service platform that allows users to easily access their data through the web. Data Lake is a single system that stores all the data, regardless of its source.
2. Self-service access to Data
The Data Lake platform provides self-service access to data for analytics and machine learning applications, but it doesn’t have an integrated query language like SQL or HiveQL (which is a query language used in the Hadoop ecosystem).
3. Minimal security
It is not recommended that you store sensitive data in this type of environment because it lacks security features such as encryption at rest and in motion; therefore, only store non-sensitive information like marketing campaign results or product catalogues.
Importance of a Data Lake
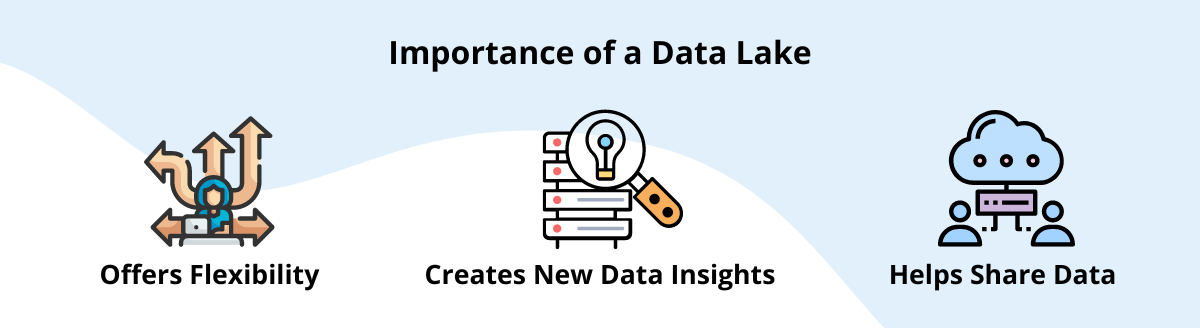
1. Offers flexibility
The main value of a data lake is that it allows you to do more with your data than you could with a traditional data warehouse.
2. Creates new data insights
A data lake lets you create new insights by combining different types of information from different sources.
3. Helps share data with other companies
It lets you share data with other companies without worrying about how they will use it or who will see it. This means that if there’s something interesting in your data that another company wants or needs, they’ll be able to get access easily—and vice versa!
Importance of a Data Warehouse
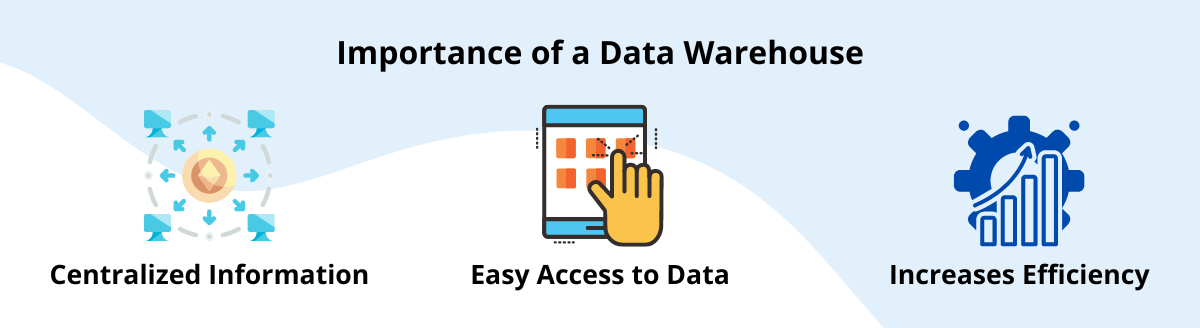
1. Offers centralized information
Centralizing all this information will allow employees to make more informed decisions based on more accurate information than ever before.
2. Easy access to data
They’ll have access to trends over time, which will allow them to predict future outcomes and make plans accordingly. Additionally, they’re easier to access than other types of databases because they offer higher performance rates than others (like SQL servers or NoSQL servers).
3. Increases efficiency
It will increase efficiency within your organization by saving time spent searching through multiple sources (like spreadsheets or emails) to find what someone needs.
Read More: What is a Data Warehouse? A Complete Guide
Key Difference Between the Data Lake and Data Warehouse
- Data Lake is a repository for storing and accessing large data sets in the form of raw data or unstructured data. Whereas Data Warehouse is a repository for storing and accessing data in a structured form.
- A data lake can be made up of multiple types of data—both transactional (like customer purchases) and descriptive (like customer demographics). But the data warehouse typically only contains transactional data.
- Another difference is that the data warehouse is often used for analysis and reporting purposes, while the data lake may be used for those purposes as well or instead.
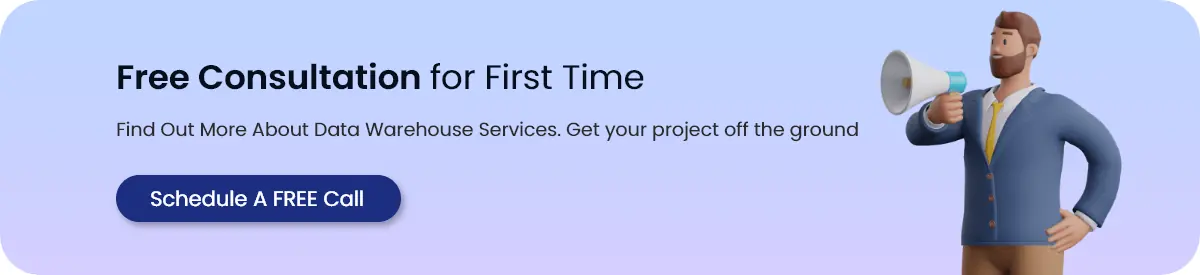
Wrapping up
The current buzzword in the business intelligence industry is “data lake”, and “data warehouse”. They both have a lot of benefits.
We hope you enjoyed learning the key differences between a data warehouse and a data lake. Remember that both can be used for business analytics, but they are not interchangeable!
FAQs Data Lake vs Data Warehouse
If you’ve ever wondered whether it’s better to use a data lake or data warehouse, I’ve got good news: the answer is yes. Having both types of systems in place can be highly beneficial.
Here are some FAQs on both of them.
A Data Lake is a new way to store, organize and access data. It’s a large repository that contains all the raw data that your business collects in one place. A Data Lake provides easy access to all your data—unlike a traditional Data Warehouse, which is designed to store only structured data.
– A Data Lake is ideal for companies that have a lot of unstructured data, such as images or video files.
– A Data Lake would be used if you need to analyze all your raw data instead of just the structured data from your database.
– It can also help you find insights in real-time by making it easier to search through all your unstructured content, such as emails or text messages from customers.
Both a data lake and a data warehouse have the same goal: to store and analyze your company’s data. However, they function in very different ways.
In a nutshell, A data lake is an all-purpose storage area that can hold any type of information—including unstructured, semi-structured, or structured—with no limitations on how long it stays there. A data warehouse holds only specific types of structured data for long periods.
You can use both. A data lake is a great place to store unstructured or semi-structured data like receipts, invoices, and contracts; however, you may want to consider also using a data warehouse for structured data such as inventory lists and customer orders.
You should also use a data lake if you need to analyze the information in near real-time (e.g., for fraud detection) or if it isn’t something that needs updating regularly (e.g., historical sales figures).
If you need historical reporting on certain metrics over time (sales trends over several years), then using an analytics platform with an online analytical processing engine might be better suited for those purposes than storing everything in your lake without any further processing.